Unlocking the Potential of Synthetic Data: How Generative AI is Shaping the Future
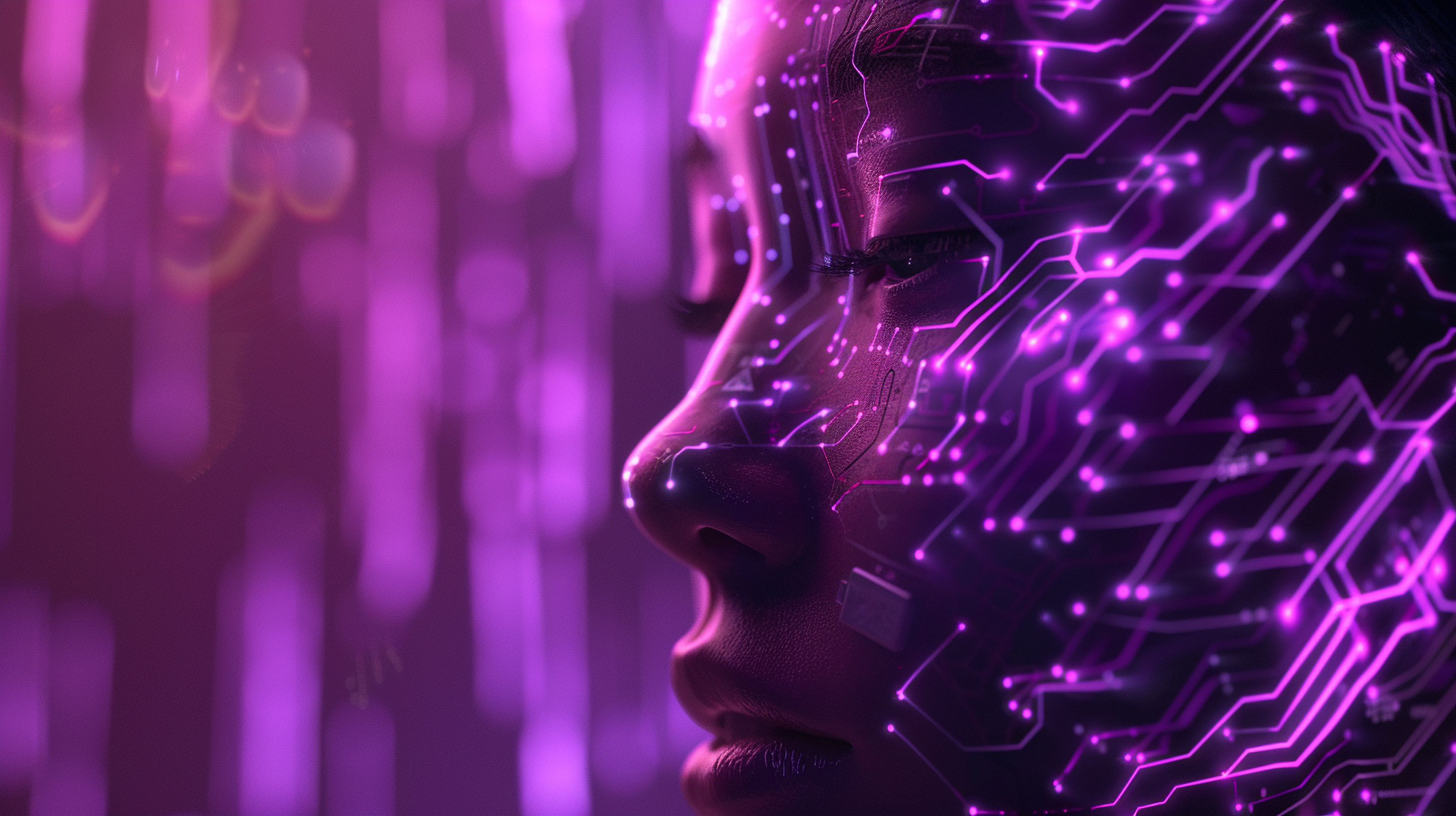
In the evolving world of data science, synthetic data is emerging as a great resource, particularly as organizations struggle with data privacy, availability, and diversity. Synthetic data is redefining how businesses across various industries approach data-driven decision-making. At the front of this revolution is generative AI, a powerful tool capable of producing high-quality synthetic data at scale, offering solutions to some of the most pressing challenges in data management and analysis.
What is Synthetic Data?
Synthetic data is artificially generated rather than collected from real-world events. Unlike anonymized data, which is actual data where personally identifiable information is removed, synthetic data is entirely fabricated but still retains the statistical properties of the original datasets. This makes it incredibly valuable for various applications, from training machine learning models to testing software systems in environments that simulate real-world conditions.
For example, in the healthcare industry, where patient privacy is a significant priority, synthetic data can train AI models without compromising sensitive information. Similarly, in financial services, synthetic data allows for rigorous testing of fraud detection systems without exposing accurate customer data.
Generative AI: The Engine Behind Synthetic Data Creation
Generative AI (particularly LLMs like GPT) revolutionizes text synthetic data creation. These models can generate realistic, diverse datasets that cover a wide range of scenarios and conditions by learning from existing data and producing new instances that mimic the characteristics of the original data. This capability is especially beneficial when accurate data is scarce, expensive to collect, or fraught with privacy concerns.
In the retail industry, for instance, generative AI can create synthetic customer text data that reflects various purchasing behaviors and preferences, enabling more accurate market segmentation and personalized marketing strategies. This not only enhances customer engagement but also helps retailers optimize inventory management by predicting demand more accurately.
Why Generative AI is a Game-Changer for Synthetic Data:
1. Data Privacy and Security: Generative AI ensures that the synthetic data it produces does not contain any accurate personal information, thus mitigating the risks associated with data leaks. This is crucial for industries like healthcare and finance, where data privacy is non-negotiable.
2. Scalability: Generative AI can quickly produce vast amounts of synthetic data, which is particularly useful for training AI models requiring massive datasets. This scalability also allows businesses to simulate multiple scenarios, improving the robustness of their models.
3. Cost-Effectiveness: Collecting and labeling real-world data can be expensive and time-consuming. Generative AI reduces these costs by automating the creation of high-quality synthetic data, making it a cost-effective alternative.
4. Bias Reduction: Synthetic data generated by AI can help address biases present in real-world data. By carefully controlling the data generation process, businesses can ensure a more balanced representation, which leads to fairer and more accurate AI Models. This means that the synthetic data can be manipulated to reduce or eliminate any biases that might be present in the original data, ensuring that the AI models built on this data are fair and accurate.
Applications Across Industries
The potential applications of generative AI for synthetic data are vast and varied:
- Healthcare: Besides protecting patient privacy, synthetic data can simulate rare diseases, enabling researchers to develop treatments for underrepresented conditions in traditional datasets.
- Finance: Synthetic transaction data can train fraud detection models, allowing financial institutions to improve security without exposing accurate customer information.
- Retail: By generating synthetic customer profiles, retailers can test new marketing strategies and inventory systems in a risk-free environment, leading to better decision-making.
- Technology: Tech companies can use synthetic data to stress-test software and hardware systems under various conditions, ensuring they perform reliably in the real world.
- Consultancy: In the consultancy industry, synthetic data can be used to model client-specific scenarios, such as market conditions, customer behavior, or supply chain disruptions. This allows consultants to test various strategies and solutions in a simulated environment. This enables them to provide more tailored, data-driven recommendations without risking client confidentiality or relying on potentially biased real-world data.